Many organisations are running very complicated human operations (thousands of ways to receive work, processes to run, systems to operate). This is not getting easier, it is getting harder. This since organisations are moving away from selling relatively simple products to far more complicated client solutions delivered concurrently across multiple channels. At the same time, as it is getting easier to interact, customers are interacting far more than previously, leading to growth of both volume and complexity.
Increase in volume, connections between channels, products, individuals, businesses and processes provides a significant scaling challenge across the growing mesh of connections that is getting increasingly complex to operate and impossible to cost effectively automate.
How do we manage operational delivery systems that are dealing with large amount of change, are getting too complex to fully understand, which depends on systems which fails in unpredictable ways and which are impossible to control using traditional approaches?
We need to start thinking differently.
We typically consider our processes as a “production chain” where items move along from creation to completion in a similar vein as what we can see in a physical manufacturing operation. However, in services we are processing data not physical goods, and physical goods has many limitations which we don’t have with data. Taking best practice from manufacturing is still not sufficient to ensure that we are getting best outcomes when handling services.
When we design or redesign a process we do “lets walk through a case” and unsurprisingly we end up with designs which are optimised for single cases. These designs are based around how the work was done on paper. We talk about customer “files”, “cases”, “folders”, “inbox” etc.
However, the way we process volume and the way we process one off transactions are inherently different. When we process volume we would like to process transactions “by type” – e.g. all transactions with the same patterns gets the same treatment and we design for those patterns. If we can do that, then we potentially become volume independent and our efficiencies become exponentially higher. When designing for high volume we typically disintegrate tasks into smaller units of work.
This is typical how software solutions gets created, with particular modules handling particular tasks with extreme efficiency. Unfortunately, the “case” or “single flow” approach we use for human process design and which is embedded in our systems today are not built for that, but rather bundling activities so that it can all be handled in “one screen” - holding us back rather than helping us forward.
Data Centric Processing is redefining the way we think about process and consider all processes only as “data creators / data consumers”. By considering the work people do from the perspective that it ultimately ends up with “data” we can tap into the rich toolsets available to handle data from “big data” platforms, for instance using machine learning to “autocomplete” the data creation when there is a pattern in what is being done. Maybe equally important, the approach also helps identify “outliers” – e.g. when people are deviating from patterns and handling something special.
As we are looking at data, we can ensure that we process all similar data elements in one go at the same time (e.g. validate an address), we can all work at the same dataset at the same time. This enable us to go beyond the processing line metaphor and a more relevant consideration is that we are aligning to what is happening in a Formula 1 Pit Stop. As soon as the work comes in, everyone can work on their areas at the same time.
The F1 pit stop is organised differently than a standard production line, but they do the same work, just far faster. More expensive you might think, but that is as they are servicing so few cars – if they had enough cars to service their superior efficiency would result in far lower cost to serve.
We can use this approach not only to be much more efficient and effective in the way we do the work, but also to really improve the service we are offering to customers. This is materially increasing ability to be competitive from a speed, cost, quality and customer service perspective – all in one go by doing things smarter.
The Data Centric approach is founded on a simple insight gained from deploying scaled robotic process automation as well as more traditional automation solutions in real world execution of high volume/high variability human processing areas. People should not do the work – they should create the datasets which enable the work to be done on underlying systems. It is far more efficient and aspirational for our people to have talent deployed to ensure the correct and timely assembly of data and to have the actual execution undertaken through Robotics, APIs or Interfaces.
To make these changes possible we need to deal with the complexity challenge which comes when we scale this approach out from a single process to an enterprise level – and that is what the Data Centric Processing approach does.
For more details on the topic, watch our on demand webinar - Time to move from Process Focused Automation to Data Centric Automation, with Simen Munter, Customer Advisory Board Member, Mindfields (previously with Nordea , ANZ and HSBC) and Mohit Sharma, Founder and Executive Chairman, Mindfields.
To stay up-to-date on Mindfields Global news, follow us on LinkedIn and Twitter.
Topic: Blog, Data Centric Automation
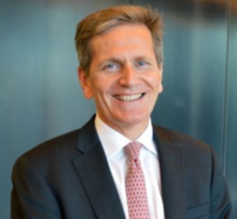
Simen Munter
Simen Munter, former Chief Operating Officer of Personal Banking at the Nordics leading retail bank, Nordea and formerly head of offshore delivery centers at ANZ Bank, has joined the Mindfields Advisory Board. He has been one of the early adopters of automation. His team consist of more than 8000 humans and 600 robots working 24 X 7. Currently more than 400 processes has been automated by these Man-Machine workforce. He was earlier CEO at HSBC and held various senior positions in HSBC.